AI can use human perception to help tune out noisy audio
In a breakthrough discovery, scientists have unveiled a novel deep learning framework poised to revolutionize audio fidelity in real-world contexts.
This advancement hinges on a previously overlooked asset: the intricate nuances of human perception.
By harnessing subjective assessments of sound quality, researchers have merged these insights with a speech enhancement model, culminating in markedly superior speech fidelity as gauged by empirical benchmarks.
This innovative model surpasses conventional methodologies in its adeptness at mitigating the intrusion of noisy audio—extraneous disturbances that often obfuscate desired auditory content.
Notably, the predictive quality scores yielded by the model exhibit a strong alignment with human judgments, underscoring its efficacy.
Traditionally, efforts to attenuate background noise have relied on AI algorithms to segregate noise from the desired signal.
However, these objective measures frequently diverge from listeners’ discernments of speech intelligibility, notes Donald Williamson, co-author of the study and an associate professor in computer science and engineering at The Ohio State University.
Williamson elucidates, “What sets our study apart is the utilization of perceptual cues to inform the model’s noise reduction mechanism.
If certain attributes of the signal are perceptible to individuals in terms of its fidelity, our model can leverage this information to refine noise suppression.”
Published in the esteemed journal IEEE/ACM Transactions on Audio, Speech, and Language Processing, the study zeroes in on augmenting monaural speech enhancement—namely, speech originating from a single audio channel, such as a lone microphone.
Drawing from two datasets compiled from prior research, the model undergoes rigorous training using recordings featuring human dialogue amid various background disturbances, including television or music. Listeners rate the speech quality of each recording on a scale ranging from 1 to 100.
The model’s efficacy stems from a collaborative learning approach, amalgamating a specialized speech enhancement module with a predictive model capable of extrapolating the mean opinion score akin to human evaluators’ judgments of noisy signals.
Results attest to the superiority of this novel methodology, showcasing marked enhancements in speech quality across perceptual quality, intelligibility, and human ratings metrics.
Nevertheless, the incorporation of human perception into audio quality assessment presents its own set of challenges, as elucidated by Williamson.
“The evaluation of noisy audio is inherently subjective, contingent upon individual auditory acuity and experiences,” he remarks.
Factors such as the utilization of hearing aids or cochlear implants further complicate matters, influencing one’s perception of their auditory milieu.
Given the pivotal role of enhancing the fidelity of noisy speech in advancing hearing aids, speech recognition systems, speaker verification technologies, and hands-free communication systems, bridging these perceptual disparities assumes paramount importance to ensure user-centric solutions.
Related Posts
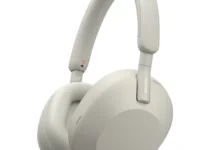
Sony’s almighty noise-canceling WH-1000XM5 headphones plummet close to their lowest price ever — this could be the perfect time to grab some AirPods Max alternatives
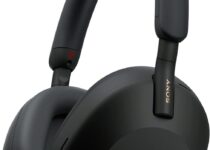
Sony’s best noise cancelling headphones are $72 off right now
